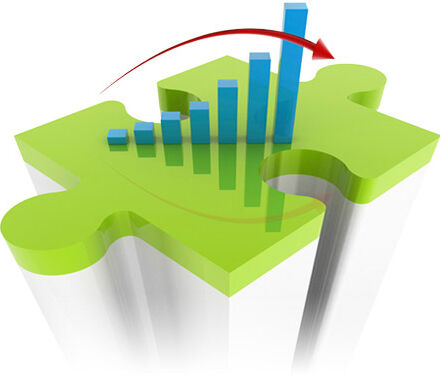
MASIM
MARKET SIMULATION
THE PRODUCT
MASIM is our tool for conducting simulations based on conjoint data. Alongside all common choice algorithms it also includes a comprehensive range of additional functions, for example for product optimization and the adjustment of models.
FEATURES IN BRIEF
- Data can be accepted from all forms of conjoint studies
- Simulation of any desired scenarios based on the defined products and prices (incl. product variations)
- Calculation of shares, sales volumes, turnover, profits and cost
- Integrated adjustment of data and models for distortion free adaptation to real market situations
- Fast optimization of individual products or product portfolios even with billions of combination possibilities
- Automatic optimization by shares, sales volumes, turnover, profits and cost
- User oriented handling despite complex options
OBJECTIVE
The data from conjoint studies offers the possibility to define market scenarios and simulate the resulting effects.
Conjoint analyses in general deliver individualized data on utility structures for each individual respondent. Thus they provide the basis for detailed calculations of purchasing behavior among the target group.
In contrast to the statistical tabulation and presentation of the average preference structures of the total sample or subsamples, in market simulations the data is applied dynamically. In the framework of the defined model, attributes, levels and products can be combined in any way in order to create new scenarios.
Thus, from a starting point that it is made as realistic as possible, the effects of variations in prices and attributes as well the impact of new product introductions on market shares, volumes, sales and profits can be tested.
METHODOLOGY
Based on the attributes and attribute levels defined in the conjoint model, a set is generated consisting of different products. The procedure then tests how each individual respondent would react if exactly this set of products were on offer in the market. In the simplest case, it tests which product is most often preferred – this is called the First Choice model.
For each respondent, this model calculates a so-called total utility value for each product – this is the sum of the utility values of the particular attribute levels that make up the product. The First Choice model assumes that the respondent will purchase the product with the highest total utility value.
When this procedure is carried out for all respondents, it is possible to calculate for each product in the set how high its share of purchase will be within the total sample.
The Share of Preference procedure splits up the purchasing preferences of each individual respondent. Unlike the First Choice algorithm, this procedure does not assume that each individual will make a clearly defined choice, but rather that the likelihoods of selecting particular products are based on the distribution of their total utility values. The individual likelihoods of selection are cumulated across all respondents and used for the calculation of market shares.
This procedure leads to smaller confidence levels, but has weaknesses when the products within a set are similar. The resulting shares of such products are unrealistically high.
An alternative procedure combines the advantages of both of the above-mentioned methodologies: Randomized First Choice. Like the Share of Preference procedure it does not assume a clearly defined choice by each respondent, but assumes different likelihoods of selecting the available products. The procedure simulates this probabilistically-determined purchasing behavior by repetition of the individual purchasing act. With each repetition, random values are added to the total utility values. The selection decision within each step is then made with the aid of the First Choice rule.
The confidence levels with this methodology are substantially lower than with a pure First Choice procedure. And the danger of overestimating similar products, as in Share of Preference, does not arise if the model parameters are chosen carefully.
Simulation models can be extended with external parameters. Thus, for example, it is possible to define costs, so that – in addition to utility values, market shares and sales – profits can also be projected.
Suitable procedures make it possible to automatically determine optimal products and product constellations. However the number of combinations to be evaluated can quickly increase to astronomical figures, so that the computing time required even by a powerful computer is no longer acceptable. Genetic algorithms can approach the optimum in a less time-consuming way, but have no necessary claim to completeness.
ADJUSTING THE MARKET MODEL
The definition of a valid model comprising attributes and their levels is an important prerequisite for achieving robust simulation results. But even the best market model cannot take account of all external effects such as differences in distribution, awareness or sales channels.
Therefore it may be useful to introduce adjustments into the market model. For this purpose, a starting scenario is defined that corresponds as closely as possible to the real-world market situation. The degree to which the result of the simulation agrees with the real-world market shares in this starting scenario is a criterion for the quality of the model. But an exact agreement is unlikely to be possible.
To achieve such an agreement, and thus to create the basis for realistic simulations, in the first place the parameters of the choice algorithm should be optimized. In most cases this enables the simulated starting point to be aligned very closely to the real market situation without distorting the data.
In further steps, specific changes can be made to the data to specifically compensate for the external effects.
PRACTICAL IMPLEMENTATION
Market simulations provide the opportunity to simulate “what if…?” situations. They offer a virtual preview of the future. They thus represent an effective and comparatively low-cost instrument in the design and pricing of products and services.
It is not necessary that the criteria to be tested must actually exist. It is only important that the respondents in the test situation are given a realistic picture of the relevant aspects. Where innovative aspects are being modeled, the simulation measures the rate of first-time purchases. In order to make valid measurements of the rate of repeat purchases, a sufficiently long phase of contact with the previously unfamiliar criteria must be inserted.
Simulations deliver concrete and very detailed projections of market constellations – even down to the number of units sold and the profits achieved. However, as with all statistical analyses, these can only offer an exact reflection of reality up to a certain point. Depending on the complexity of the defined scenarios, the size of the sample, the respondents’ answering behavior and many other aspects, the actual behavior of the target group will deviate from what has been projected.
A particular danger of this methodology is the failure to take account of this statistical uncertainty in the interpretation of the results.
Due to volume of calculations involved, simulations can only be carried out with the aid of computer programs. Such programs differ widely in their scope and operation. For the implementation of simulations, IfaD offers the online tool MASIM. This includes all established choice procedures, and permits adjustments to market starting-point situations, profit projections and product optimizations with both genetic and full algorithms.
The tool comprises a flexible user and rights administration module, so that users only have access to those settings that are relevant for them.